Can everyone be above the average?
By Salvatore A DellaVilla Jr., CEO, Strategic Power Systems Inc.
I remember a professor relating an observation attributed to Mark Twain to get the class thinking about data and how they are used statistically. He said, “Statistics don’t lie, but liars figure.” My professor got the desired laughter and went on to say that while there is some tongue-in-cheek with this jeu de mots, there also is an element of truth in it. The message: When looking at someone else’s data, beware—or at least be careful.
In the world of powerplant data and performance evaluation, RAM (Reliability, Availability, Maintainability) metrics have real economic value—up front as the project is defined and financed, and over time, as the plant operates through its lifecycle.
RAM metrics are used to establish pro forma project viability by creating a high degree of confidence that an acceptable rate of return will be achieved. They also are used to justify plant improvement through upgrade opportunity, with improved economics, extended life, and increased profitability.
Additionally, RAM metrics are used in trade literature and industry technical programs to inform the market that expectations for “high reliability” not only will be met, they will be exceeded—an important message, especially as technology advances with higher output, improved efficiency, and lower emissions.
Finally, they are used to communicate what best-in-class performance looks like in a competitive market. OEMs and owner/operators are driven to be best-in-class, to achieve the highest levels of RAM performance. RAM metrics do make a difference; they have value and they are indicative of equipment performance. But remember the professor’s message.
A toss of the coin: Is that what RAM is?
I always have had a tough time accepting availability, reliability, starting-reliability, and service-factor numbers of 98% and higher (I cringe at 99%). My gut reaction always is, “Really?” That’s normally followed by, “Over what period of time and for how many units or plants?”
Additional questions usually will include the following:
- Does the time period reflect a full maintenance cycle?
- Which technology class of units?
- What operating duty cycle(s)?
- What fuel(s)?
- What frequency?
- Simple cycle or combined cycle?
My goal is not just to challenge, but to understand, to build a level of confidence—statistical confidence—so I’m sure the numbers have real meaning and value. It’s called stratifying or segmenting the data to characterize what it represents. I think my professor would have been pleased with this approach.
My perspective is that 98% means success 98 times out of 100 tries; conversely, failure just 2% of the time or twice in 100 attempts. If you toss a fair coin looking for a head, that means you can’t get there in only 10 tosses; nine out of 10 is only 90% success. To get to 98%, you would need a minimum of 50 tosses with 49 of those resulting in a head. What do you think the probability of that is?
Think for a moment about the challenges associated with achieving 98% starting reliability on a fast-start peaking gas turbine. It means that the sequence of actions from start initiation, to ignition, to flame, to ramp-up in speed, to synchronous speed, to breaker closure, to achieving a sustainable load must happen successfully 98 times out of 100—typically within 10 minutes. Now consider the even greater challenge of achieving 98% starting reliability on a combined-cycle unit.
If you think of success or failure in terms of time, 98% success would mean 8584.8 uptime hours (service plus reserve) or just 175.2 downtime hours (planned and unplanned maintenance plus forced outages). A key performance independent variable is the mission or the demand profile that must be satisfied—peaking, cycling, or base load.
In today’s market, the economic incentive is to be available, generating and making money at the spark-spread price; or in ready reserve with associated capacity payments. The objective is to maximize uptime, to be available and ready for dispatch—98% of the time for the purposes of this discussion.
Another approach to achieving the uptime objective is to minimize downtime. Note that 175.2 hours of annual downtime is not much—just over one week (168 hours). The next question: What equipment is included in the plant statistic? This depends on the type of plant, simple- or combined-cycle and, if the latter, whether it is single- or multi-shaft.
Achieving 98% combined-cycle availability is a formidable task given the number of components (steam turbine, HRSG, feedwater pumps, etc) and parts, the levels of required maintenance based on operations (hours and/or starts), and unexpected outages. The frequency of outage events and the time to repair/restore must be managed very effectively.
Relative to downtime, bear in mind that plant economics drive how expeditiously maintenance and repairs are completed. Maintenance intervals and times to perform normally are determined by the duty cycle or mission profile in terms of maximizing the readiness to serve. To illustrate: A peaking plant does not necessarily have the same incentive as a cycling or base-load plant to perform maintenance in the optimum timeframe with the maximum workforce and associated number of shifts.
Maintenance intervals and times to perform are a logistical issue that uses time and resources as efficiently as desired, and that depends on plant goals and objectives—and whether an availability or reliability bonus/penalty arrangement exists. If there is an economic incentive to increase annual availability or reliability by 1%—that is, by an additional 87.6 hours of uptime—then management control will be applied to achieve this financial objective or opportunity.
Does this mean that 98% is not achievable? No, it can be achievable; but for how long, for how many units, and through what O&M cycle? Is it a one-off unit or one-off plant, is it an average unit or plant, or is it best-in-class? I believe that a well-designed plant with cost-effective O&M practices is the primary requirement for meeting high reliability or availability goals, whatever the numbers.
It is not like the toss of a fair coin. The probability of success is not random and not just 50%. It depends on plant design, the level of component redundancy, and the ease of maintenance and other logistical issues. Nonetheless, the challenge is to understand what the numbers represent: average, median (middle of the road), mode (most common value); or, perhaps, the maximum (best-in-class).
Distribution and variability
When viewing data, normally you are looking at a distribution of performance: the good, the bad, the ugly. If you perceive the data points as a distribution and think of the 98% value, what does it mean? How much variability is included in the distribution? Is it the average, the mode, the medium, or were you lucky enough to be best-in-class.
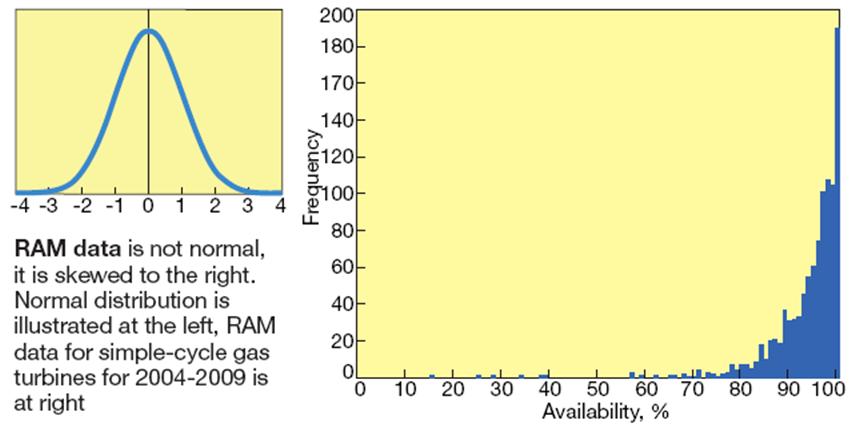
If the last, keep in mind that best-in-class means repeatable and sustainable performance through the major maintenance cycle of the unit and/or plant—not just once or for one week, month, or year. Minimizing variability is critical to achieving the highest levels of RAM performance—a six-sigma approach. If the data distribution is highly variable or highly skewed then the unit-to-unit or plant-to-plant performance variability may be too high.
The charts illustrate the difference between the normal bell-shaped curve and the skewed characteristic of RAM data. Minimizing variability assures that even the average plant has a level of RAM performance that is relatively consistent with about 70% of the other plants. This equates to a well-designed plant employing cost-effective O&M practices.
Note that data distribution is just that, a range of values reflecting varying levels of performance. The measures of central tendency—the average, median, mode, maximum, minimum, and standard deviation—provide the tools for understanding the distribution of values. For both the OEM and owner/operator alike, there is a strong desire, driven by market expectations, to be Number One—to believe that they have the highest levels of RAM performance when compared to others.
And virtually everyone has the same message: “We’re the best!” However, for those with a vested interest, it is important to recognize that not everyone is above or better than the average value. That’s impossible. And far fewer are best-in-class. In fact, best-in-class normally is a moving target.
Regardless of what company news releases would have you believe, there are bad performers, average performers, and above-average performers. The desire to portray data in the most favorable light sometimes blurs the fine details of a complex picture, fitting in nicely with another maxim from Mark Twain, “Get your facts first, then you can distort them as you please.”
Understanding the 98% performance measure requires an engineering assessment of the data presented to determine statistical accuracy, significance, and validity. First step: Verify that the data are random by confirming that there has been no pre-selection of units or plants for either inclusion or exclusion from the sample (data pool) based on RAM performance.
The sample must be indicative of the performance of the general population so market inference can be drawn with a high degree of belief that the assessment is statistically meaningful. Plus, outliers in the data—that is, extremely high or low RAM values—must be identified and excluded from further analysis.
The statistical process described is the correct approach. If the analysis is not rigorous and conducted in this manner, then we are back to where we started: “Statistics don’t lie, but liars figure.” And without an inquisitive approach to the data, Caveat emptor.
Analyzing real data
To put this discussion further into perspective, let’s look at RAM performance data from two independent sources: NERC GADS and ORAP®. The data from both systems is similar in structure and content, but may come from different plants of varying technologies, duty cycles, and ages.
NERC GADS is the North American Electric Reliability Corp’s Generating Availability Data System. It is empowered by the Federal Energy Regulatory Commission (FERC) and focuses on the generating mix and on generation RAM performance at the highest levels to ensure the reliability of our country’s bulk power system.
By contrast, the Operational Reliability Analysis Program (ORAP), is a third-party system operated and maintained by Strategic Power Systems Inc (SPS), which focuses primarily on gas- and steam-turbine-powered plants arranged for simple- and combined-cycle service. It provides much greater detail than GADS at the component and system levels for benchmarking and engineering analysis, as well as for design-for-reliability processes.
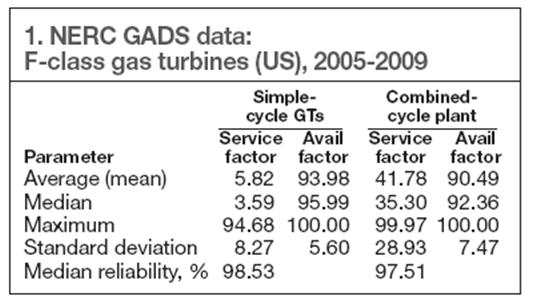
Table 1 displays the most current data (2005-2009) available from GADS for F-class gas turbines and combined-cycle plants (US only). To simplify the data assessment, only two RAM metrics are provided for consideration: service factor and availability factor. For each, the statistical measures of central tendency are provided—average, median, maximum, and standard deviation—along with the median reliability (includes only forced and unplanned outages). These statistics are plant measures. Note: No data, including outliers, have been culled from the data set.
The GADS data clearly show that 98% availability is neither average nor middle-of-the-road (median). It is approaching best-in-class. Other important observations include the following:
- The average service factor for simple-cycle GTs, 5.82%, is greater than the median (3.59%). This implies that the data set is skewed to the left—that is, the majority of the individual unit-year data values are below the average. These units clearly are in peaking service. On average, they are in reserve standby at least 8250 hr/yr.
- The standard deviation around the availability factor for each data set is of interest. These values are relatively small, indicating a low level of variability around the mean. The larger standard deviations around the service factor indicate a higher level of plant-to-plant variability.
- Combined-cycle availability is 90% to 92%, with units (both single- and multi-shaft) in service around 3480 hr/yr, based on a review of the service factors.
- Median reliability is reported as approaching 98%—that is, 50% of the individual unit-year data are above the achieved value, 50% below. These values may indeed reflect expected performance. However, the correct allocation of downtime is an important question that would require further assessment.
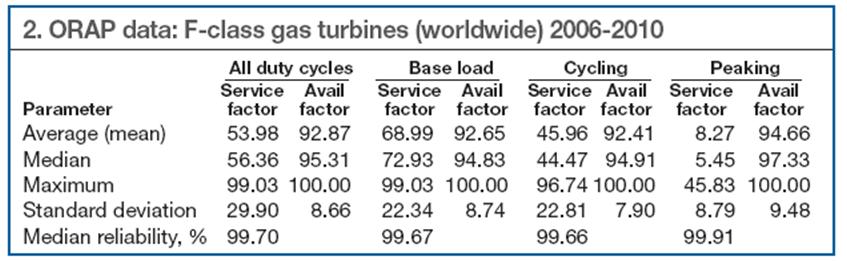
Table 2 presents F-class 2006-2010 ORAP data for participating units worldwide (both 50 and 60 Hz. It is consistent with GADS data and the metrics are representative of the plants. ORAP RAM data are shown for all duty cycles combined, as well as by individual duty cycle, to illustrate the impact of the operational paradigm on plant availability.
In today’s market, operational flexibility is a requirement—specifically, fast start, load following, and high availability. These metrics should assist in formulating appropriate expectations for equipment performance based on the way the plant is intended to operate and be maintained. It is useful for pro forma input. An important metric that normally would be included in this assessment is starting reliability.
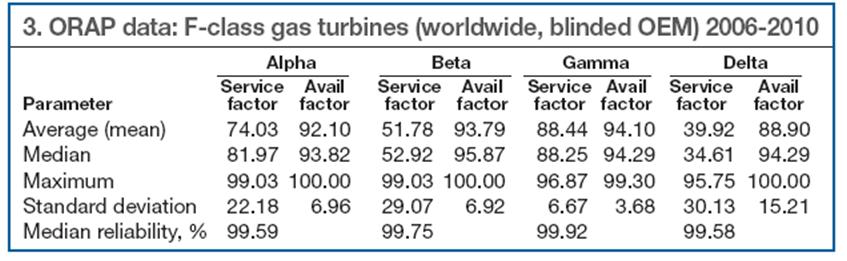
Table 3 demonstrates that not everyone is above the average, as noted earlier. There are clear differences in service-factor and availability data. Of particular interest is the standard deviation around the availability factor to understand the spread around the mean for each OEM, labeled Alpha, Beta, Gamma, and Delta.
Note: The data for Gamma represent relatively few unit-years and the data set is more homogeneous than the others because it represents higher-duty-cycle plants—as evidenced by the service factor with a tight standard deviation.
So, the reality is there are differences in RAM performance. It is just as important to recognize that there is a difference between average or even median performance and best-in-class. And what often is communicated at industry meetings in terms of RAM metrics is more likely best-at-the-moment than best-in-class.
We all want to be best-in-class, we all want to be above the average, but it is important to understand what a true benchmark value of average is—because the majority of the fleet is closer to the average or median. Just look at the availability metrics. Only the maximum values meet or exceed the 98%, and they normally would be treated as outliers statistically. The reliability metrics meet or exceed the 98% value, and they should be investigated more closely to understand why.
ORAP data collection, review, validation process
Since 1987, SPS has processed and entered in its ORAP® database plant operational, failure, and maintenance data representing over 22,000 unit-years of service and more than 280,000 forced, scheduled, and unscheduled outages. Information from over 2000 individual gas and steam turbines is streamed to SPS monthly for review and validation.
These data provide the energy industry RAM benchmarks for both heavy-duty (frame) and aeroderivative plants across the various OEMs, technologies, applications, and duty cycles. Information is received from a variety of sources, including plant O&M and headquarters operations personnel. In some cases, operating data—not outage details—are received directly from the unit control system or onsite historian.
Regardless of the source, no plant data can be added to the ORAP database until it passes both a Manual Data Validation (MDV) and an Automated Data Validation (ADV) process. Industry standards, such as IEEE 762 and ISO 3977, underpin the validation processes.
Each piece of data received is reviewed by an SPS customer service engineer with a focus on reasonableness and technical accuracy. Any questions that arise in the data must be addressed directly with the participating plant’s representative as soon as possible.
In fact, an SPS customer service engineer is in almost constant contact with plant staff to address questions and issues with the information provided, or to address questions and issues the plant may have regarding the data or ORAP RAM metrics provided to them. SPS takes full responsibility for the accuracy and the quality of reported data and spends the engineering time required to achieve the highest level of quality possible.
There is a significant emphasis placed on all reported outages—forced, scheduled, and unscheduled—down to one-tenth of an hour. When assessing forced outages, the objective is to clearly understand the symptom, corrective action, and eventually the root cause of failure. In terms of scheduled or unscheduled maintenance, the emphasis includes the time to perform and the frequency of maintenance compared with recommended OEM practice.
SPS engineers analyze information from all outages to ensure that the assignment of a standard equipment code, at a system and component level, is accurate and reflects the performance of the specific technology. EPRI Standard Equipment Codes are used in this effort.
There are many ADV rules for automatically reviewing and assessing data for accuracy. However, only after completion of the MDV process can plant data be submitted for inclusion in the ORAP database. Customer reports cannot be issued until the responsible—read accountable—customer service engineer passes reviewed and approved plant data to the reporting database. The objective is to manage and contain data discrepancies, and to ensure reporting accuracy.
The SPS data review and validation process is rigorous and time-consuming. But, it is critical to achieving the level of quality demanded by the industry. It is essential that the review of field data be performed by knowledgeable engineers who understand plant equipment and have a strong attention to detail. This is what makes ORAP a unique and value-added information resource.
Trust, but verify
Many in the industry may remember the words “trust, but verify.” They date back to the late 1980s, a Reganesque mantra used to drive home the necessity of validating the mutual destruction of all intermediate-range ballistic missiles by theUSSRand theUS. These words were on the motto of the On-Site Inspection Agency, established in 1988 within the Dept of Defense. The goal was right for everyone, but the proof was in the inspection and verification process.
I know RAM metrics are not of a nuclear caliber, but they do have force on our industry. SPS often is questioned about the current RAM statistics when they are presented at conferences. The discussion normally starts out something like, “I just sat through a technical presentation by all the OEMs and they all presented their current RAM metrics. . . .”
Then the bomb is dropped: “Can everyone really be above the average?” It is a fair question, but I do get a headache when I hear it several times throughout the day. We all know that everyone is not above the average. The real question is, “Who is below the average?”
Consequently, it’s time that SPS take a similar approach of “trust, but verify,” to give the electric-power and energy industries a higher degree of confidence that ORAP RAM metrics have statistical accuracy, validity, and are defensible. To this end, SPS now provides a Certificate of Verification to indicate that its statistics have been vetted and approved for presentation by its reliability engineers.
This stamp of approval means that SPS stands behind the statistics being presented and that the data used to develop the metrics are available for review. You might consider this as similar to a UL certification for industry RAM data. Bear in mind that the information presented might not be from ORAP at all; it may come from another source or internal system. But if it’s not ORAP information, it will not have the certificate of verification. CCJ